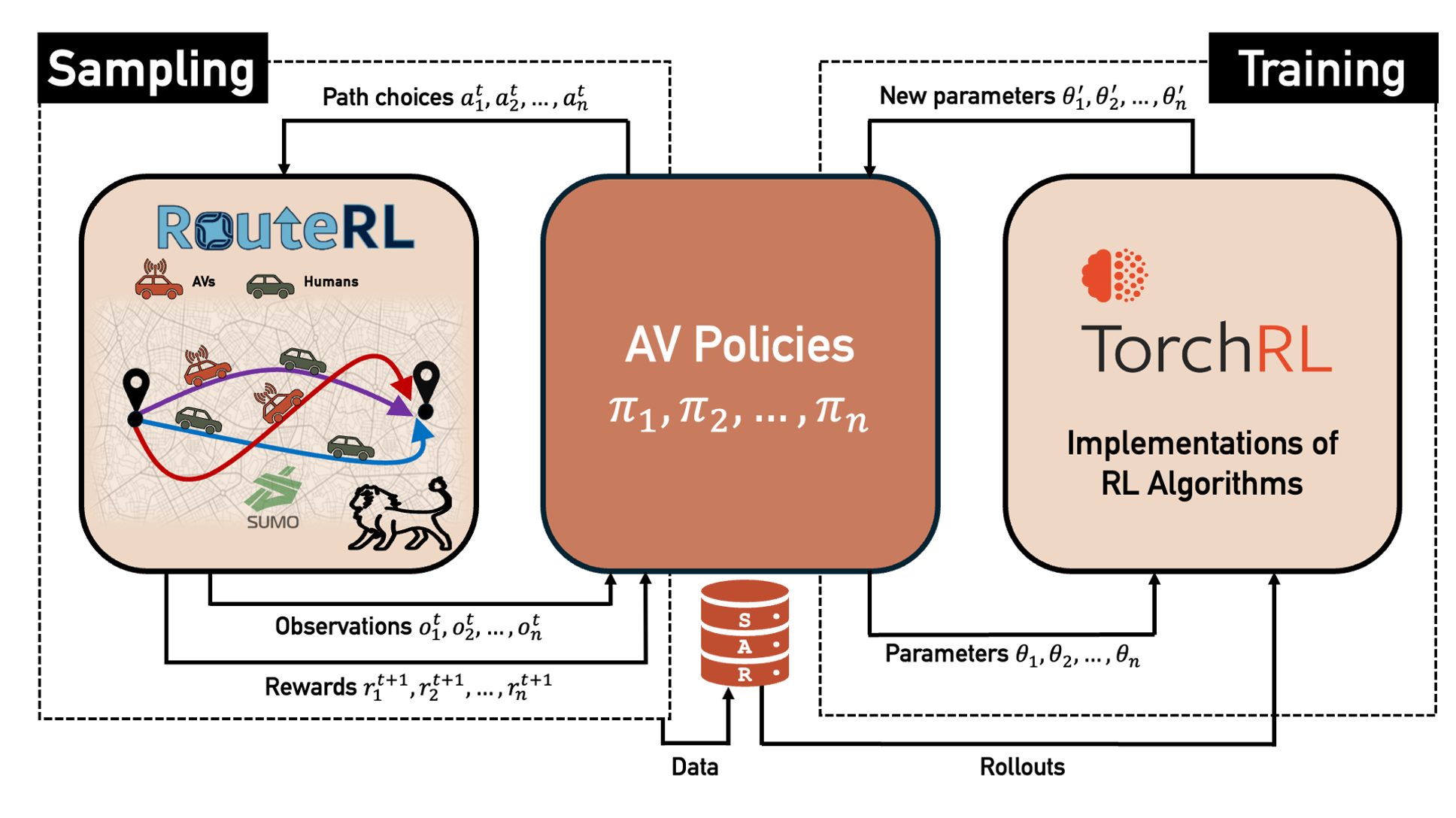
RouteRL is a novel framework that integrates Multi-Agent Reinforcement Learning (MARL) with a microscopic traffic simulation, SUMO, facilitating the testing and development of efficient route choice strategies. The proposed framework simulates the daily route choices of driver agents in a city, including two types:
human drivers, emulated using discrete choice models,
and AVs, modeled as MARL agents optimizing their policies for a predefined objective.
RouteRL aims to advance research in MARL, traffic assignment problems, social reinforcement learning (RL), and human-AI interaction for transportation applications.